Beginning in 1972, a team of researchers at IBM began to reorient speech recognition from the study of language and perception towards a startling new mandate: “There’s no data like more data.” Systematically prying speech recognition away from the simulation of symbolic reason and linguistic processes, the IBM Continuous Speech Recognition group refashioned it as a problem of purely statistical data processing. In doing so, they spurred the development and diffusion of data-driven algorithmic techniques that are today standard throughout speech and natural language processing, and increasingly pervasive in knowledge practices across the humanities and the sciences alike. This project examines how speech recognition, as a problem of reconciling acoustic measurement with linguistic meaning, helped bring language under the purview of data processing as something that could not only be formatted and stored digitally, but also analyzed and even interpreted algorithmically. It traces the pivotal role of such efforts to computationally map sound to language in shaping the conceptual, industrial, and technical foundations that gave rise to the proliferation of “big data” analytics, machine learning, and sibling algorithmic practices across diverse domains of knowledge production and into the sphere of everyday life.
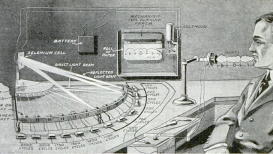
An illustration depicting the envisioned design of the "phonoscribe", a voice-operated typewriter proposed by John B. Flowers. Source: Darling, Lloyd: The Marvelous Voice Typewriter: Talk to It and It Writes. In: Popular Science Monthly, July 1916.
Project
(2017-2019)